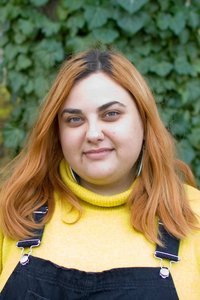
PhD student
MRes Ioanna Kotari
T
+43 1 25077-4329
E-Mail
During my undergraduate studies at the University of Crete in Greece, I specialised in Environmental Biology and the management of natural resources. I completed my thesis on paralogous gene evolution and expression and I continued on an internship at University College London, where I dove into learning how to use bioinformatics skills to study substitution models in deep phylogenies. This internship sparked my interest in developing more computational skills to handle evolutionary questions and led me to obtain an MRES degree on “Computational methods in ecology and evolution” at Imperial College London. My master’s thesis focused on developing a deep learning algorithm to distinguish between hard and soft selective sweeps from low coverage sequencing data of non-model organisms.
During my PhD project, I will be expanding on polymorphism-aware phylogenetic models (PoMos) by introducing PoMo-cod, a codon substitution model for detecting signatures of natural selection on protein-coding genes. PoMo-cod will allow to disentangle the effects of natural selection from known confounding forces (such as mutational biases, demography and GC-biased gene conversion) in determining the genetic diversity of multiple populations and species, thus producing more accurate genome-wide maps of diversifying evolving genes.