- Home /
- University /
- Infoservice /
- Press Releases /
- High-tech animal protection: mini-sensors for gentle control of wild boar
Research
High-tech animal protection: mini-sensors for gentle control of wild boar
So-called accelerometers are commercially available as ear tags. In principle, this enables automated, low-disturbance recording of animal behavior - a great benefit for behavioral studies, but also for wildlife management. However, these sensors often only scan at a low frequency, e.g. one Hertz. A recent study at the Research Institute of Wildlife Ecology (FIWI) at the University of Veterinary Medicine, Vienna, therefore examined the extent to which this very low data acquisition rate in wild boar allows the calibration of various behaviors.
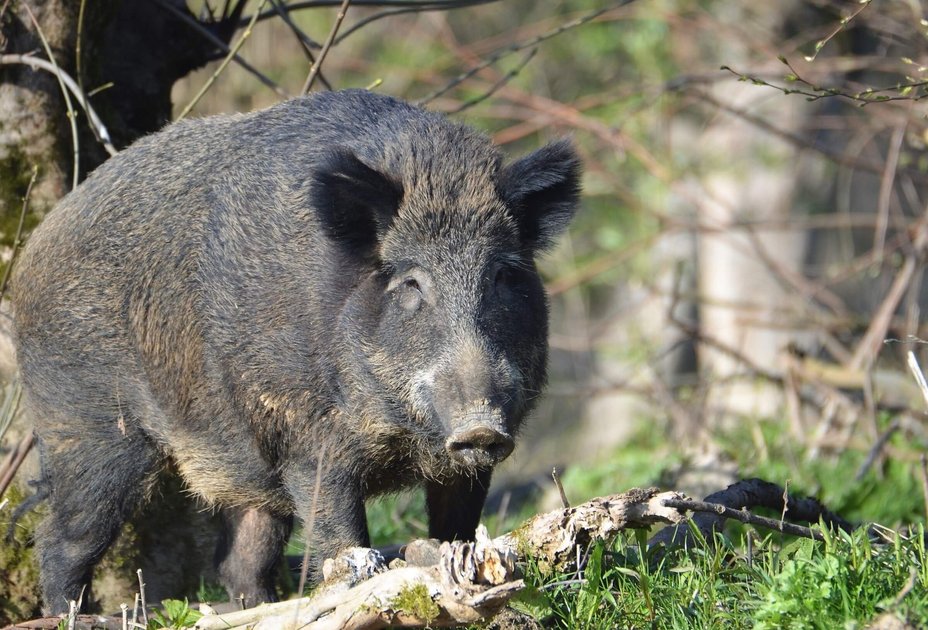
In their study, the scientists classified the behavior of female wild boars kept under semi-natural conditions in a large outdoor enclosure using acceleration data. The behavioral predictions were based on a machine learning algorithm, in particular the random forest model in the open source software h2o. According to Thomas Ruf from the Research Institute of Wildlife Ecology (FIWI) at the Vetmeduni, first author of the study, this combination paid off: “Remarkably, the calibration and thus also the prediction of many behaviors was possible with the tiny acceleration sensors on the ear tags, even though they only scan the data at a low frequency.” According to Ruf, these miniature measuring devices are particularly suitable for significantly extending the shelf life of the battery in the ear tag and thus minimizing the potentially harmful effects of repeatedly capturing wild animals to replace batteries.
Population control of wild boar as a possible field of application
According to the researchers, the long battery life of the mini-sensors will also help to collect long-term acceleration data, for example to research seasonal trends. Foraging, resting and suckling of young animals were well recognized, while standing and walking, for example, were not reliably detected. Study last author Sebastian G. Vetter: “The accuracy depended on the type of behavior and ranged from 50 % for walking to 97 % for lateral resting. To predict the behavior, the algorithm used static features of the unfiltered acceleration data and the filtered gravity and orientation data. However, the waveform of certain behaviors in the sampled frequency range did not play an important role.”
Machine learning revolutionizes the use of accelerometers
Larger variants of accelerometers have been used for several decades in many animal species to quantify their behavior. The purpose of the measurements in up to three axes is to identify animals as active or resting, to calculate approximate values for their energy consumption or to classify the behavior of animals as foraging or running, for example. The identification of behavior based on body acceleration requires advanced analysis techniques such as principal component analysis, discriminant analysis or - especially recently - machine learning (ML) algorithms. Thomas Ruf comments: “The advent of ML has greatly facilitated the use of accelerometry data to classify behavior. This is a real revolution, especially for the use of mini-sensors.”
The article „Classification of behaviour with low-frequency accelerometers in female wild boar“ by Thomas Ruf, Jennifer Krämer, Claudia Bieber and Sebastian G. Vetter was published in „PLOS One“.
Scientific article
Scientific contact:
Öffentlichkeitsarbeit und Kommunikation
Veterinärmedizinische Universität Wien (Vetmeduni)
medienanfragen@vetmeduni.ac.at